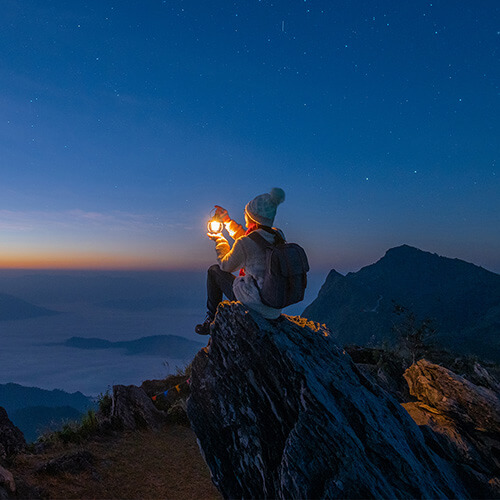
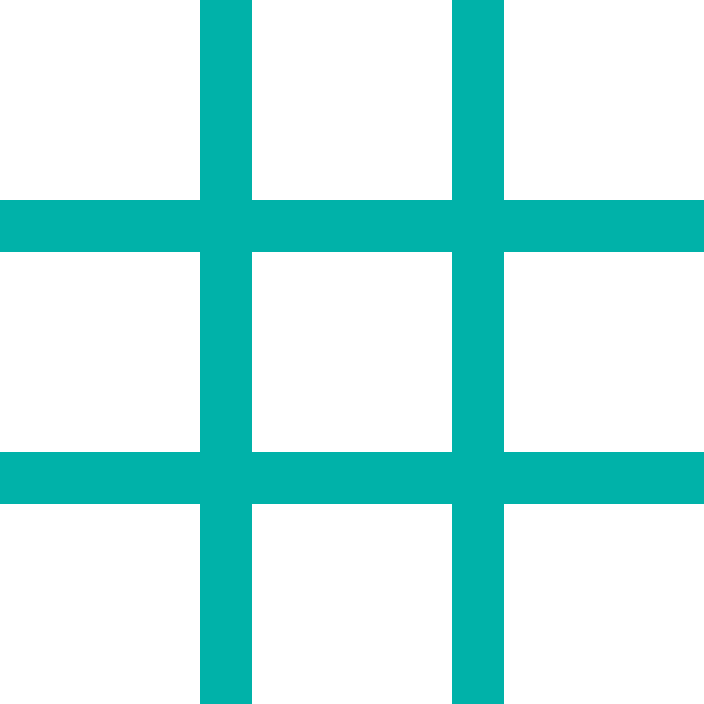
Looking to hire or need leadership advice?
Get in touch to discuss your executive search or leadership development needs.
Find a consultant Make an inquirySubscribe to our global magazine to hear our latest insights, opinions, and featured articles.
For any leadership challenge, we have the solution.
From detailed executive searches to leadership coaching, we provide a recruitment service that can help your organisation advance with confidence.
Get in touch to discuss your executive search or leadership development needs.
Find a consultant Make an inquiry